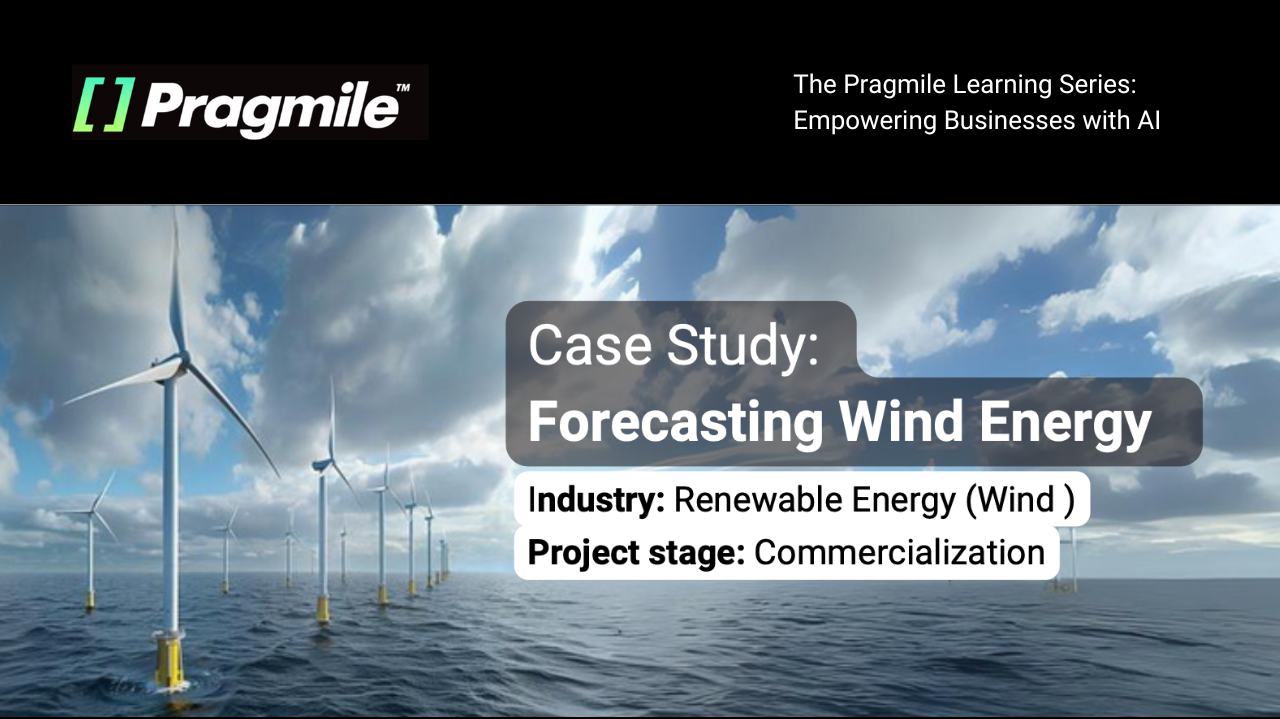
Case Study: Forecasting Wind Energy
Introduction
A startup called SOLAR SPY, which is now a thriving business, faced increasing demands from clients who also operated wind energy systems. These clients experienced the same manual processing issues with wind data as they did with PV data. To address this, Solar Spy partnered with Pragmile to develop an advanced wind energy forecasting tool, including automated DNO (Distribution Network Operator) reporting services.
Our Achievements:
- Developed a comprehensive AI-driven forecasting tool specifically for wind energy.
- Improved forecast accuracy, reducing the error margin for neural network models by up to 6%.
- Automated manual data processing, significantly enhancing operational efficiency.
- Ensured regulatory compliance with evolving EU regulations.
Client Benefits:
- Provided precise metrics on wind energy production and forecasting accuracy.
- Enhanced operational efficiency by automating data input and processing.
- Ensured compliance with EU energy regulations with minimal manual intervention.
The Problems:
Solar Spy’s clients faced several challenges:
- Manual Data Processing: Their existing systems relied heavily on manual data input for wind energy, which was time-consuming and prone to errors.
- Regulatory Compliance: Keeping up with evolving EU regulations for energy production and reporting was complex and resource-intensive.
- Forecast Accuracy: Existing models did not provide the required precision for wind energy forecasting, leading to operational inefficiencies and potential financial losses.
Our Solution:
Pragmile proposed developing a robust, AI-driven forecasting tool specifically for wind energy, along with automated DNO reporting services. This tool was designed to enhance prediction accuracy and streamline data processing, addressing the key challenges faced by Solar Spy’s clients.
Path to Success:
- Data Verification and Analysis: The first step involved verifying and analyzing accessible data to ensure its accuracy and relevance, which was crucial for reliable forecasting and insights.
- Prototype Development and Integration: Pragmile developed and integrated a prototype of the advanced forecasting tool. This prototype utilized deep neural networks to enhance prediction accuracy, trained with weather and production data.
- Implementation and Feedback Collection: The tool was implemented and continuously refined based on feedback from Solar Spy, ensuring it met their specific needs.
- Advanced AI Model Training: The advanced AI models are currently being further trained and will be fed back into the system soon to accommodate upcoming 15-minute forecasting frequency requirements.
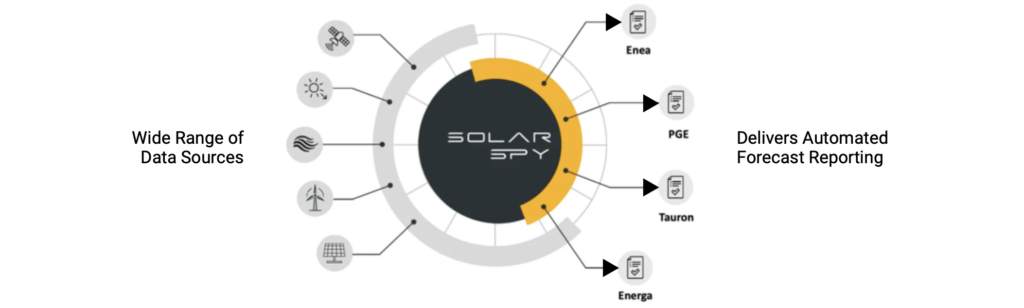
Results:
- Improved Forecast Accuracy: The new forecasting tool significantly improved prediction accuracy, reducing the error margin for neural network models by up to 6%.
- Operational Efficiency: Automation reduced manual data processing, saving time and reducing the likelihood of errors.
- Regulatory Compliance: The system was designed to adapt to EU regulations, ensuring Solar Spy and its clients remained compliant with minimal manual intervention.
Conclusion:
Solar Spy’s journey with Pragmile highlights the critical role of advanced analytics in transforming renewable energy forecasting. By leveraging AI and machine learning, Solar Spy significantly improved forecast accuracy and operational efficiency for both PV and wind energy. This case study demonstrates the potential for similar companies to harness advanced technologies to drive innovation and efficiency in the energy sector.
Key Takeaways from Solar Spy’s Journey:
- User Feedback Integration: Actively incorporating user feedback is crucial for optimizing functionality and user satisfaction.
- Scalability of Tools: Building scalable tools ensures long-term viability and the ability to handle increasing data volumes and complexity.
- Regulatory Adaptability: Designing systems that can easily adapt to regulatory changes minimizes compliance risks and operational disruptions.
What Pragmile Offers:
- As Pragmile continues to push the boundaries of innovation, businesses that partner with us can expect to benefit from their deep expertise, cutting-edge technologies, and commitment to delivering bespoke solutions. By harnessing the power of AI, Computer Vision, and Machine Learning, Pragmile empowers organizations to build a future where ideas meet expertise, driving transformative results across industries.
Empowering projects with our High-Performance Developer Teams. - Harnessing the power of AI, Computer Vision, and Machine Learning to craft pioneering solutions.
- Utilizing our Infrasenses technology makes tech solutions smart, intuitive, and predictive.
- Creating robust AI-driven platforms and developing bespoke tech solutions tailored to your needs.
Download the full Case Study here > Link.
Schedule a free consultation with
our AI and technology experts
Take advantage of the latest AI solutions, tailored to your company's needs. Book a consultation with AI solution architects at Pragmile and discover new opportunities in energy management.
Please, provide your business email to schedule a meeting