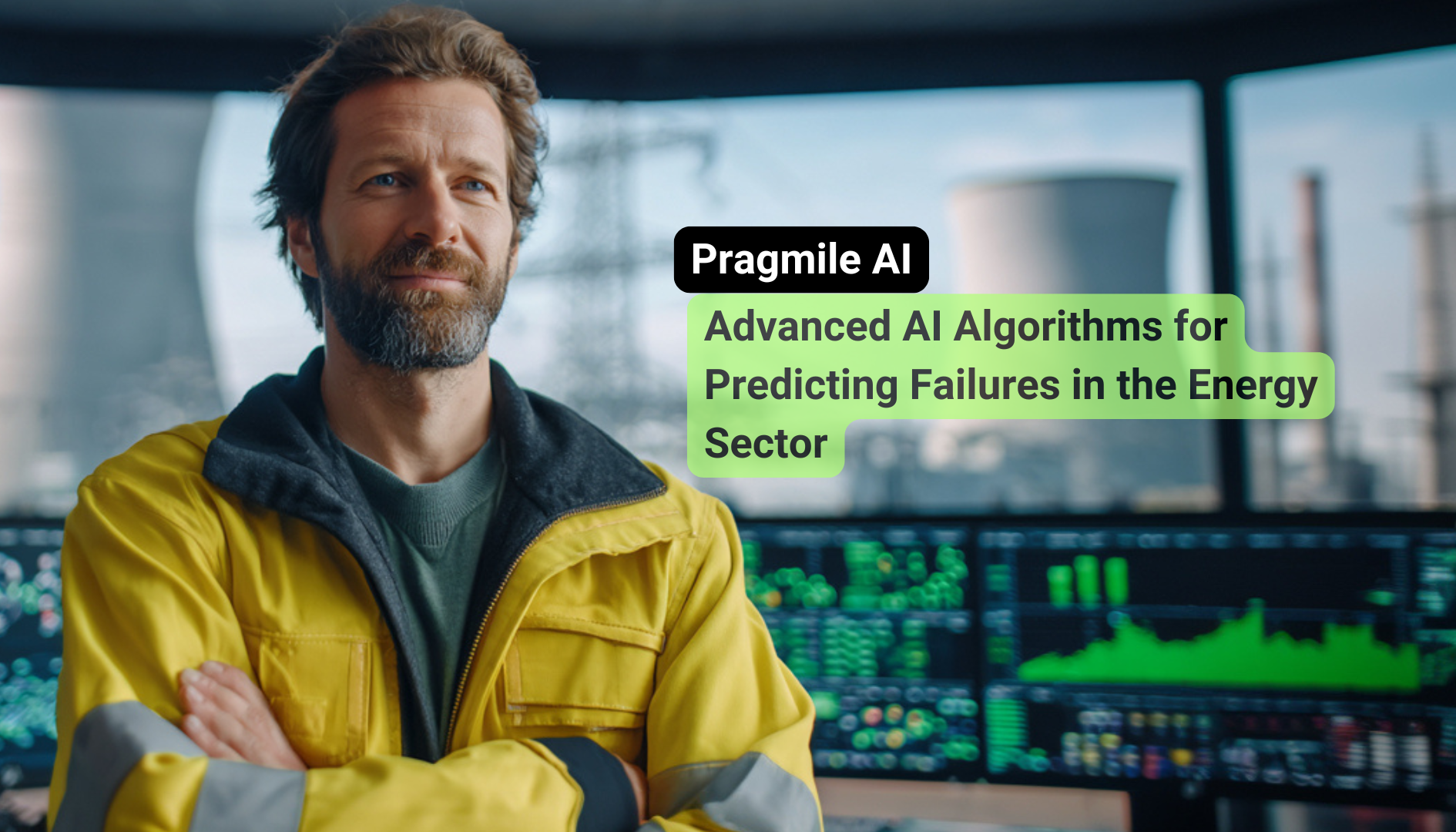
Published: November 20, 2024
Advanced AI Algorithms for Predicting Failures in the Energy Sector
In the energy industry, infrastructure failures lead to serious consequences. Downtime in power plants or problems in energy transmission not only generate high costs, but can also lead to negative effects on the economy and public safety. A solution that is gaining popularity is the use of artificial intelligence (AI) to predict failures. Predictive maintenance is an advanced tool that, with the help of predictive analytics, enables reducing the risk of failures, increasing machine efficiency, and improving operational safety.
Energy Failures – a Challenge for the Energy Sector
In the energy sector, industrial failures are one of the biggest operational challenges. Power plant failures can cause large-scale power disruptions, leading to significant financial losses and problems for energy consumers. Power outages can be the result of both problems with key infrastructure elements and difficult-to-predict mechanical damage. For this reason, energy companies are constantly looking for modern methods of monitoring equipment and methods of predicting energy failures to prevent unplanned power outages.
The traditional approach to maintenance is based on regular inspections and technical reviews, but in the face of the increasing need for effective infrastructure management, this is not enough. Modern solutions such as predictive analytics and predictive maintenance are increasingly used, which allow detecting potential breakdowns long before they occur.
Predictive maintenance is an approach that uses data to predict failures and plan preventive actions. In the energy sector, these predictive maintenance techniques are extremely important. Examples of predictive maintenance applications include monitoring the condition of equipment and analyzing data on their performance. This helps minimize risks such as power plant failures and increase operational efficiency.
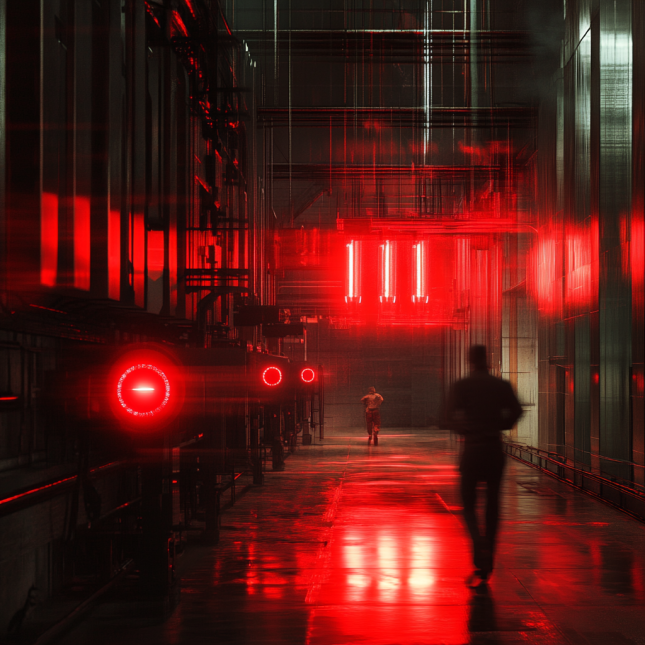
AI for Energy: Artificial Intelligence in Failure Forecasting in the Energy Sector – Role and Benefits
Artificial intelligence in the energy sector is a field that has been developing very dynamically in recent years. The value of the global AI market in the energy sector was $13.18 billion in 2023. It is estimated that in 2024 it will be $15.45 billion, and by 2034 its value is to amount to approximately $75.53 billion.
Using AI to forecast failures enables monitoring of data from sensors and analysis of device operation patterns, which allows for identification of deviations from the norm. On this basis, it is possible to predict where and when a failure may occur.
Advanced AI algorithms used for predictive maintenance are able to analyze huge amounts of data from many sources – from sensors installed on machines, through SCADA systems, to historical data. Thanks to artificial intelligence, it is possible to more effectively monitor the condition of devices, predict failure moments and optimize maintenance schedules.
The benefits of using AI in energy management include the following:
- Increasing System Reliability – thanks to early detection of failures, AI helps minimize the risk of energy supply disruptions.
- Optimization of Maintenance Costs – planned repairs are much cheaper than urgent failure removal.
- Improving Work Safety – AI can detect potential threats to employees, e.g. overheating of devices.
- Increasing Energy Efficiency – AI algorithms can optimize the operation of devices, which leads to reduced energy consumption.

AI Algorithms Used to Predict Energy Failures
Artificial intelligence uses various algorithms to precisely analyze data and predict failures. The most important AI algorithms used in the energy sector include the following:
- Machine Learning (ML) – ML models are able to recognize patterns based on large data sets, e.g. they can predict when a failure will occur, based on the analysis of failure history and sensor data.
- Deep Learning – deep learning neural networks are advanced tools that can process complex data, such as images from cameras monitoring the condition of machines. Thanks to this, they can detect the smallest deviations from the norm.
- Predictive Analysis – based on patterns, predictive analytics allows for the prediction of technical problems before they occur. When combined with AI, it is one of the most advanced solutions in failure risk management.
- Probabilistic Algorithms – based on historical data, they create models that calculate the probability of failure for a specific component. Thanks to them, it is possible to effectively manage resources and preventively replace wearing elements.

Examples of Using AI to Predict Failures in the Energy Sector
Artificial intelligence is widely used in the energy sector – also when it comes to predicting failures. For example, implementing AI algorithms in wind turbines allows for monitoring the technical condition of each component. Based on data from sensors, AI analyzes changes in rotational speed, vibrations, and temperature, which allows for predicting failures before they occur.
Artificial intelligence also uses camera images and thermal imaging analyses to monitor the condition of transmission lines. This allows for detecting places where components are overheating, which helps avoid power outages. AI also allows for monitoring the temperature of transformers, their voltage, and other operating parameters, which allows for predicting when a failure may occur.
Below you can find some AI-based predictive maintenance examples in the energy sector.
A market example of using AI to predict energy failures is the American startup Rhizome. The company has created an artificial intelligence system aimed at predicting the probability of power grid failures as a result of extreme weather events, such as snowstorms or wildfires. The solution uses utility companies’ historical data on the performance of energy equipment and combines it with global climate models.
Another example is the I-NERGY project, which aims to create a platform supporting AI-based energy management. The prototype of the system has been tested in 9 pilot centers. The results of the pilots were promising – for example, AI-based fault analysis and predictive maintenance of circuit breakers allowed for accurate classification of incidents in about 96% of cases.
The Polish company Solar Spy offers an AI-based SaaS solution for comprehensive management of photovoltaic farms, which we at Pragmile had the pleasure of co-creating. Among other things, it allows for automatic detection of anomalies such as shading, panel contamination, or inverter failures, helping to minimize downtime and ensure continuity of energy production. The platform integrates weather data, historical performance records, and current operational indicators to predict potential failures.
The use of advanced artificial intelligence algorithms in predicting energy failures brings significant benefits to the entire industry. Predictive maintenance supported by AI allows you to reduce the risk of unplanned downtime, reduce repair costs, and ensure continuity of energy supply. Thanks to AI, the energy sector is becoming more efficient, safer, and prepared for new challenges.
If you want to delve deeper into the use of AI algorithms in predicting energy failures and find out how you can use this solution in your business, schedule a free consultation with Marcin Jabłonowski – Managing Director and AI Solutions Architect at Pragmile.
Schedule a free consultation with
our AI and technology experts
Take advantage of the latest AI solutions, tailored to your company's needs. Book a consultation with AI solution architects at Pragmile and discover new opportunities in energy management.
Please, provide your business email to schedule a meeting