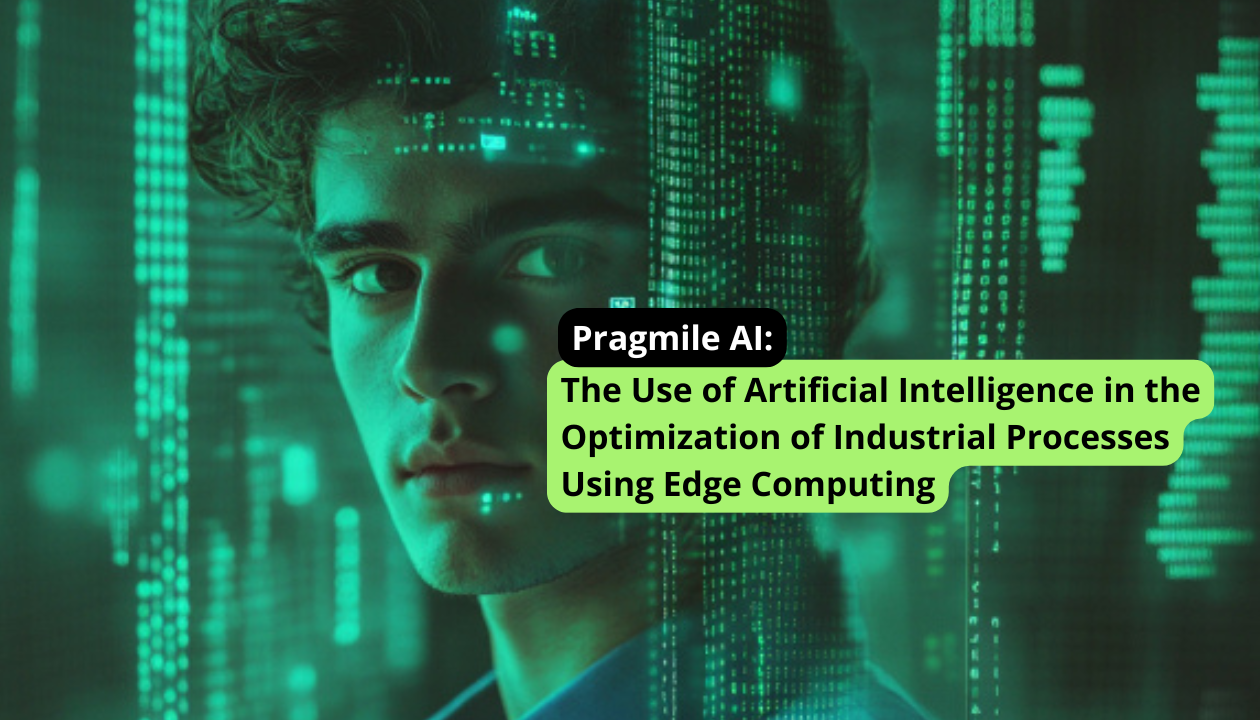
Published: December 4, 2024
The Use of Artificial Intelligence in the Optimization of Industrial Processes Using Edge Computing
Modern industry is undergoing a dynamic transformation, driven by new technologies, including artificial intelligence (AI) and edge computing. Edge computing enables data processing close to its source, which provides faster access to analysis results and greater flexibility in production management. Edge computing combined with artificial intelligence gives manufacturing companies enormous potential to increase efficiency, improve quality, optimize and automate industrial processes, as well as reduce operating costs and more.
Edge Computing and Artificial Intelligence in the Manufacturing Industry
Traditionally, data from sensors and industrial devices is sent to the cloud, where it is analyzed. However, due to the long delays in data transmission, this solution is not always sufficient, especially in the case of processes that require a quick response.
Edge computing changes this situation. It is a data processing model that moves calculations closer to the data source, instead of relying on central servers in the cloud. This makes it possible to process information faster and reduce delays, which is crucial in industrial environments. In addition, local processing supports security, eliminating the risk of sensitive data leakage.
Edge computing enables IoT (Internet of Things) devices to generate data and analyze it instantly, allowing for faster decision-making. This means that factories can analyze data from thousands of sensors and cameras in real time to improve productivity and monitor production quality.
Artificial intelligence plays an increasingly important role in the optimization of industrial processes. Artificial intelligence in production allows for the automation of many tasks, as well as predicting machine failures or optimizing the supply chain. By using machine learning algorithms, companies can analyze huge amounts of data and draw valuable conclusions from them.
Artificial intelligence embedded in IoT devices allows for real-time data analysis, anomaly detection, prediction of future events and autonomous decision-making. An example could be car factories, where AI edge computing monitors the performance parameters of robots on the production line, which allows for a quick response in case of problems.
It is predicted that by 2026, 75% of large enterprises will rely on AI-based processes to increase assets efficiency, streamline supply chains and improve product quality. By then, at least 50% of edge computing implementations are expected to include machine learning (compared to 5% in 2022). Furthermore, it is estimated that by 2027, as much as 62% of enterprise data will be processed at the edge.

Edge Computing Architecture
Edge computing architecture allows for flexible scaling of data processing systems, which enables their adaptation to the specific requirements of industrial enterprises. It consists of a network of local processing nodes that communicate with each other and with central systems, ensuring stability and minimizing the risk of delays. This architecture works perfectly with IoT and AI systems, creating complex production solutions.
With a properly designed edge computing architecture, manufacturing plants can gain a competitive advantage by responding to changing operating conditions in real time. For example, in the electronics industry, AI and edge computing can support the assembly processes of precision components, controlling quality and compliance with technical requirements. Such applications of edge computing and artificial intelligence in factories contribute to reducing waste, increasing efficiency and improving work safety.
Edge Computing and Artificial Intelligence in Manufacturing – Benefits of Combining Technologies
Integrating artificial intelligence with edge computing (AI edge computing) in an industrial environment enables more efficient and smarter manufacturing, with benefits that include both operational and strategic improvements to factory operations. These include the following:
- Real-Time Operational Optimization – edge computing enables local processing of data using AI, eliminating the need to send data to remote servers. This allows for monitoring and optimizing production processes in real time, which is especially useful for automating production lines and monitoring machine operating conditions. Examples of such applications include AI systems analyzing sensor data on assembly lines, which immediately detect anomalies, preventing major downtime.
- Predictive Maintenance and Failure Management – using AI, edge computing enables real-time analysis of machine sensor data, predicting potential failures before they occur. For example, AI algorithms can monitor vibration, temperature, or noise levels to detect deviations from the norm, giving the opportunity to replace parts or service equipment before serious problems arise. This type of predictive maintenance leads to reduced repair costs and increased equipment durability.
- Reducing Latency and Optimizing Network Bandwidth – edge computing minimizes the delays associated with sending large amounts of data to the cloud or central servers. This is important when the factory needs to react quickly to changing production parameters, as in the case of systems for monitoring the quality of products on production lines. Local processing also helps reduce the load on the network infrastructure, which is especially important when supporting extensive IoT systems.
- Improving Product Quality and Compliance – AI supported by edge computing allows for verification of product quality even before production is completed. Algorithms can monitor various production parameters, including dimensions, colors or texture and immediately inform about deviations. Such quality control enables quick corrections, which leads to a reduction in the number of defects and an increase in the compliance of products with quality requirements, which in turn results in greater customer satisfaction.
- More Efficient Management of Energy Resources and Cost Optimization – by monitoring the energy consumption of various machines, AI can identify areas requiring optimization in real time, which allows the adjustment of the operating parameters of the devices to current needs. Automatic adjustment of resource consumption contributes to reducing operating costs and optimizing resource use, which is crucial for enterprises with large energy needs.
- Increased Work Safety – edge computing allows monitoring of the work environment and data from safety-related sensors, such as gas detectors, object detection systems or image recognition technologies. By analyzing this data, AI is able to identify potential threats and respond immediately, for example by warning employees about dangerous conditions or stopping the machine in emergency situations.
- Faster Implementation of Innovations and Shortening the Production Cycle – edge computing allows for the implementation and testing of new solutions directly on the production line, which speeds up the innovation process. AI can process data in real time, providing immediate feedback, which allows for rapid improvements and faster time to market.
- Security of Sensitive Data – edge computing enables data processing locally, directly at production devices, which minimizes the need to transfer sensitive information to the cloud or central servers. Leaving data on-site reduces the risk of leakage or unauthorized access, increasing security.

Optimization of Printed Circuit Board, Car and Tire Production Processes Using Artificial Intelligence and Edge Computing
At Siemens Electronics Works Amberg (EWA) factory, artificial intelligence and edge computing are used to optimize key production processes. The quality of soldered joints on printed circuit boards is verified by AI, which analyzes sensor data directly at the device. The algorithm predicts whether the soldered joints are correct, which reduces the number of tests and increases efficiency. But that’s not all. In the same factory, predictive maintenance is used in the spindle milling process – AI analyzes the spindle speed and current consumption parameters, predicting failures 12 to 36 hours in advance. Edge computing allows this data to be processed on site, which minimizes the risk of downtime and increases process reliability.
Audi, in turn, uses AI and edge computing to automate the quality control of welds at the Neckarsulm plant, where around 1,000 cars are produced daily. With Intel Industrial Edge Insights, the system analyzes data from welding tools in real time, which enables precise detection of defects and a reduction in the number of manual inspections. This solution allows Audi to reduce operating costs (including reducing labor costs by 30-50%) and increase automation, improving production quality, because the system analyzes data directly at the production line level.
Another example is Shanghai DeepSight, which, with the support of Intel technology, has developed a solution based on artificial intelligence and edge computing to improve defect detection in the tire production process. Thanks to real-time image analysis, the system detects small defects with an accuracy of over 99.9%, reducing the inspection time to less than 1 second. This solution allows for the inspection of over 20,000 tires per day, increasing efficiency, reducing labor costs by about $42,000 per production line, and minimizing waste.
The integration of edge computing with artificial intelligence in manufacturing is the foundation of modern production solutions, enabling companies to flexibly and effectively manage processes in real time. The benefits include not only significant improvements in quality and production efficiency, but also better control over operating costs and energy resources. AI and edge computing contribute to the development of Industry 4.0, helping manufacturing companies respond faster to changes and increase competitiveness in the global market. If you want to learn how these technologies can support your business, schedule a free consultation with Marcin Jabłonowski – Managing Director and AI Solutions Architect at Pragmile.
Schedule a free consultation with
our AI and technology experts
Take advantage of the latest AI solutions, tailored to your company's needs. Book a consultation with AI solution architects at Pragmile and discover new opportunities in energy management.
Please, provide your business email to schedule a meeting